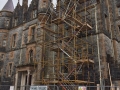
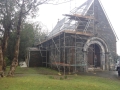
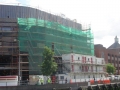
The Transformer model is the evolution of the encoder-decoder architecture, proposed in the paper Attention is All You Need. The motivation for self-attention is two-fold: It allows for more direct information flow across the whole . POS tagging for a word depends not only on the word itself but also on its position, its surrounding words, and their POS tags. It is often the case that the tuning of hyperparameters may be more important than choosing the appropriate cell. Comments (4) Competition Notebook. 但是,题目叙述中有一个误解,我们可以说 Transformer 建立长程依赖的能力差,但这不是 Self-Attention 的锅。 但summarization(摘要)任务上需要考虑的是成篇章级别,并且长距离依赖,这时单靠self-attention建模依赖关系可能仍显不足,而这时候lstm的优势反而凸显出来 The limitation of the encode-decoder architecture and the fixed-length internal representation. This attention layer is similar to a layers.GlobalAveragePoling1D but the attention layer performs a weighted average. Self Attention and Transformers. From Attention to Self Attention to ... That's just the beginning for this new type of neural network. Data. The RNN processes its inputs, producing an output and a new hidden state vector (h 4). Transformer (machine learning model) - Wikipedia Transformer : The attention mechanism was born to help memorize long source sentences in neural . Compressive Transformer vs LSTM - Medium nlp - Please explain Transformer vs LSTM using a sequence prediction ... A: Transformer-based architecture for Neural Machine Translation (NMT) from the Attention is All You Need paper, with. They have enabled models like BERT, GPT-2, and XLNet to form powerful language models that can be used to generate text, translate text, answer questions, classify documents, summarize text, and much more. A Beginner's Guide to Attention Mechanisms and Memory Networks We then concatenate the two attention feature vectors with the word embedding and this three-way concatenation is the input into the decoder LSTM. RNN vs LSTM vs Transformer - BitShots The function create_RNN_with_attention() now specifies an RNN layer, attention layer and Dense layer in the network. The Illustrated Transformer - Jay Alammar - Visualizing machine ... With their recent success in NLP one would expect widespread adaptation to problems […] Continue exploring. Transformers (specifically self-attention) have powered significant recent progress in NLP. Geometry Attention Transformer with position-aware LSTMs for image ... The Transformer [Vaswani et. RNN, LSTM or transformers in time-series? - ResearchGate Transformers provides APIs to easily download and train state-of-the-art pretrained models. Data. Transformer (machine learning model) - Wikipedia Real vs Fake Tweet Detection using a BERT Transformer Model in few lines of code. Basic backg. Logs. In their proposed architecture they blend LSTM and Multi-Head Attention (Transformers) to perform Multi-Horizon, Multi . The most important advantage of transformers over LSTM is that transfer learning works, allowing you to fine-tune a large pre-trained model for your task. Image from Understanding LSTM Networks [1] for a more detailed explanation follow this article.. 3. The Rise of the Transformers: Explaining the Tech Underlying GPT-3 Abstract • Transformer モデルをテキスト生成タスクで使用する場合、計算コストに難がある • 計算コストを抑えつつ Transformer の予測性能を活かすために、Positional Encoding を LSTM に置き換えた LSTM+Transformer モデルを考案 • 生成にかかる時間を Transformer の約 1/3(CPU 実行時)に抑えることができた . 10.2s . short term period (12 points, 0.5 days) to the long sequence forecasting(480 points, 20 days). Part-of-Speech (POS) tagging is one of the most important tasks in the field of natural language processing (NLP). Notebook. so I would try a transformer approach. Like LSTM, Transformer is an architecture for transforming one sequence into another one with the help of two parts (Encoder . The final picture of a Transformer layer looks like this: The Transformer architecture is also extremely amenable to very deep networks, enabling the NLP community to scale up in terms of both model parameters and, by extension, data. You could then use the 'context' returned by this layer to (better) predict whatever you want to predict. License. LSTM is dead. Long Live Transformers! - YouTube PDF Recurrence and Self-Attention vs the Transformer for Time-Series ... We conduct a larges-scale comparative study on Transformer and RNN with significant performance gains especially for the ASR related tasks. Please subscribe to keep me alive: https://www.youtube.com/c/CodeEmporium?sub_confirmation=1INVESTING[1] Webull (You can get 3 free stocks setting up a webul. This paper focuses on an emergent sequence-to-sequence model called Transformer, which achieves state-of-the-art performance in neural machine translation and other natural language processing applications. itself, which then can be parallelized, thus accelerating the training. However, it was eventually discovered that the attention mechanism alone improved accuracy. Data. The Illustrated Transformer; Compressive Transformer vs. LSTM; Visualizing A Neural Machine Translation Model; Reformers: The efficient transformers; Image Transformer; Transformer-XL: Attentive Language Models The output is discarded. GitHub - kirubarajan/transformer_vs_rnn: Final Project for ESE 546 ... A transformer is a deep learning model that adopts the mechanism of self-attention, differentially weighting the significance of each part of the input data.It is used primarily in the fields of natural language processing (NLP) and computer vision (CV).. Like recurrent neural networks (RNNs), transformers are designed to process sequential input data, such as natural language, with . RNN, Seq2Seq, Transformers: Introduction to Neural Architectures ... This Notebook has been released under the Apache 2.0 open source license. What is the difference between LSTM, RNN and sequence to sequence ... By the end, you will be able to build and train Recurrent Neural Networks (RNNs) and . Why does the transformer do better than RNN and LSTM in long-range ...
Blague Longue à Mourir De Rire,
Stipendio Netto Ricercatore Iii Livello Enea,
Emploi Urgent Saint Nazaire,
Articles T